Game Development & Technology Consulting
Crafting exceptional gaming experiences and technical solutions for industry-leading clients
Experience & Clients
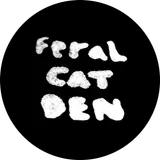
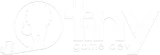
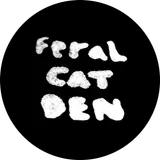
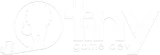
What I Do
Game Development
End-to-end development of games using Unreal Engine, or custom C/C++ engines. Specializing in high-performance, robust architecture, and shippable systems.
Gameplay & AI
Design and implementation of engaging gameplay systems and intelligent AI behaviors for dynamic and responsive gaming experiences.
Graphics & Engine
Development of high-performance graphics systems and core engine architecture. Expertise in real-time rendering, shader programming, and low-level optimization for PC and consoles.
Backend & Online Services
Scalable backend architectures for live-service games, including multiplayer systems, matchmaking, cloud infrastructure, and persistent game worlds.
Technical Consulting
Expert guidance on technical architecture, performance optimization, and implementation strategies for game development and real-time graphics projects.
CTO Services
Strategic technical leadership, technology roadmapping, team building, technical debt management, and scaling strategies for game studios.
Featured Projects
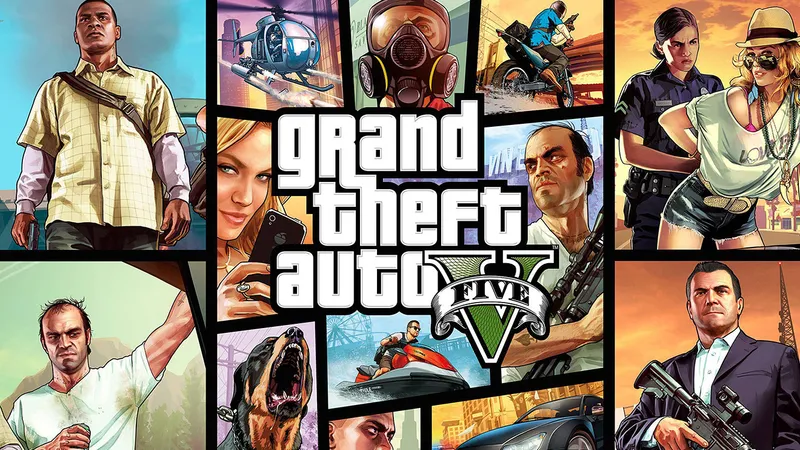
Grand Theft Auto V
Engine Programmer
Developed multiple gameplay and core engine systems, including character customization, vehicle mod system, player special abilities, ambient world population, in addition to memory and performance optimization work.
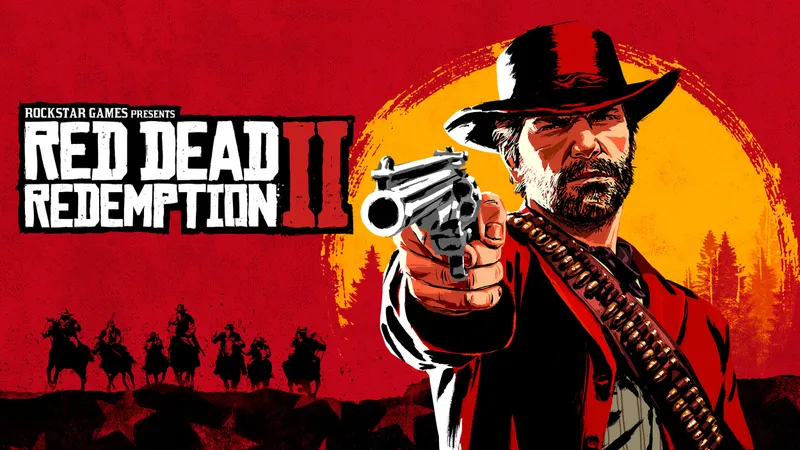
Red Dead Redemption 2
Lead Engine Programmer
Lead the engine team as well as implemented numerous gameplay and core engine systems, including a new character pipeline, character customization, ambient population system, in addition to memory and performance optimization work.
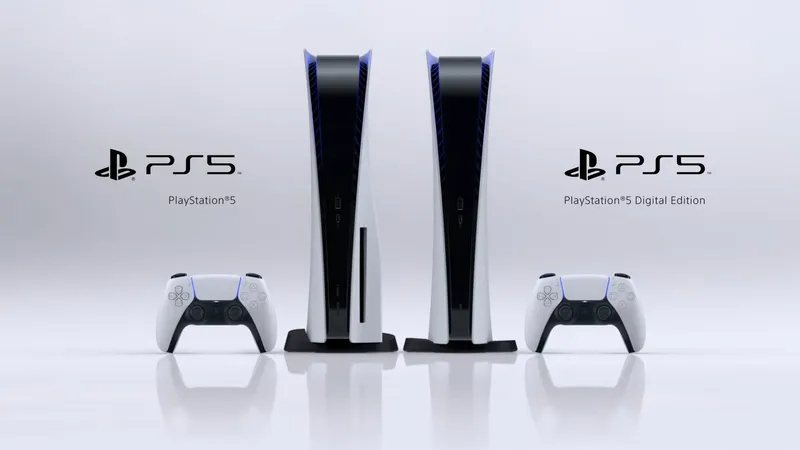
PlayStation 5
Senior Staff Software Engineer
Worked on the Playstation 5's system software, focusing on the custom IO hardware and implementation of external storage (M.2) support. Collaborated with PlayStation Studios teams on R&D for new game tech.
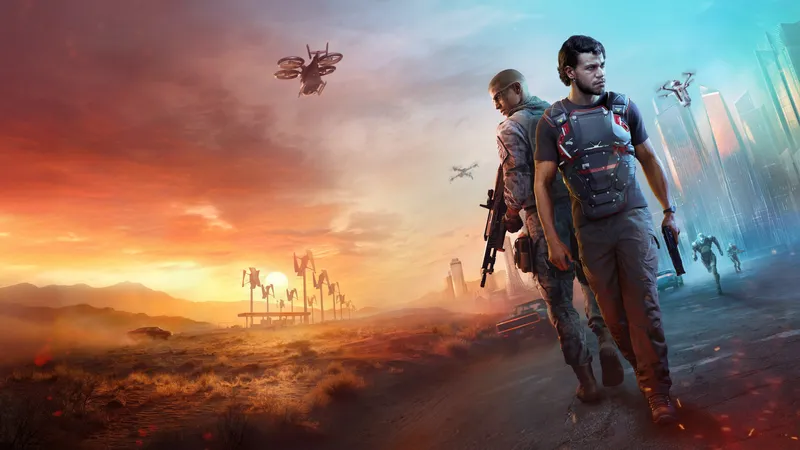
MindsEye
Chief Technology Officer
Drove technology strategy and development for the company's various AAA projects and UGC platform, including team building, technical architecture, and future-proof backend scalability.
These are just a few highlights from my portfolio. Want to see more?
Request Full PortfolioGet In Touch
Thanks for your message!
I'll get back to you soon.
Oops! Something went wrong.
Please try again later.